Activity recognition track competitors
Activity Recognizition Teams

Team name Chiba Yu-Lab |
| ||
Corresponding author | |||
Affiliation | |||
Description Our previous studies demonstrated that the idea of bio-monitoring home healthcare mobile robots is feasible. Therefore, by developing algorithms for mobile robot based tracking, measuring, and activity recognition of human subjects, we would be able to help impaired people (MIPs) to spend more time focusing in their motor function rehabilitation process from their homes. For this purpose: 1) tracking strategies for different types of home environments were tested in a simulator to investigate the effect on robot behavior; 2) a multi-channel saliency fusion model with high perceptual quality was proposed and integrated into RGB-D based visual tracking. |
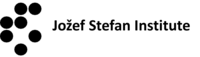
Team name JSI |
| ||
Corresponding author | |||
Affiliation | |||
Description |
Team name AReM |
| ||
Corresponding author | |||
Affiliation | |||
Description |
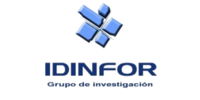
Team name AmevaActivity |
| ||
Corresponding author | |||
Affiliation | |||
Description |